Beyond Correlation: Global Courses on Impact Evaluation
This section offers a carefully curated list of courses on impact evaluation, causal statistical inference, development economics, econometrics, and general economics from the world's most prestigious universities. These courses are taught by field experts, including some Nobel laureates in economics. They are available on various online education platforms such as MITx, EdX, and Coursera.
By clicking on the images corresponding to each course title, you'll be directed to the official course webpage. In many cases, access to course materials, lectures, and exams is free. However, if you wish to obtain a certificate to validate your knowledge, course fees range from $30 to $1,000 USD. Substantial financial aid is often available for students from developing countries, and prices frequently drop from $1,000 to around $100, so don't let the costs discourage you.
Please note that we simply disseminate this valuable material and do not endorse any particular course or have affiliations with the institutions offering them. Our goal is to make this knowledge accessible to undergraduate and postgraduate students in developing countries. We want to highlight that these resources for learning impact evaluation methods, development economics, and econometrics are readily available, just a few clicks away.
Data Analysis for Social Scientists - MITx
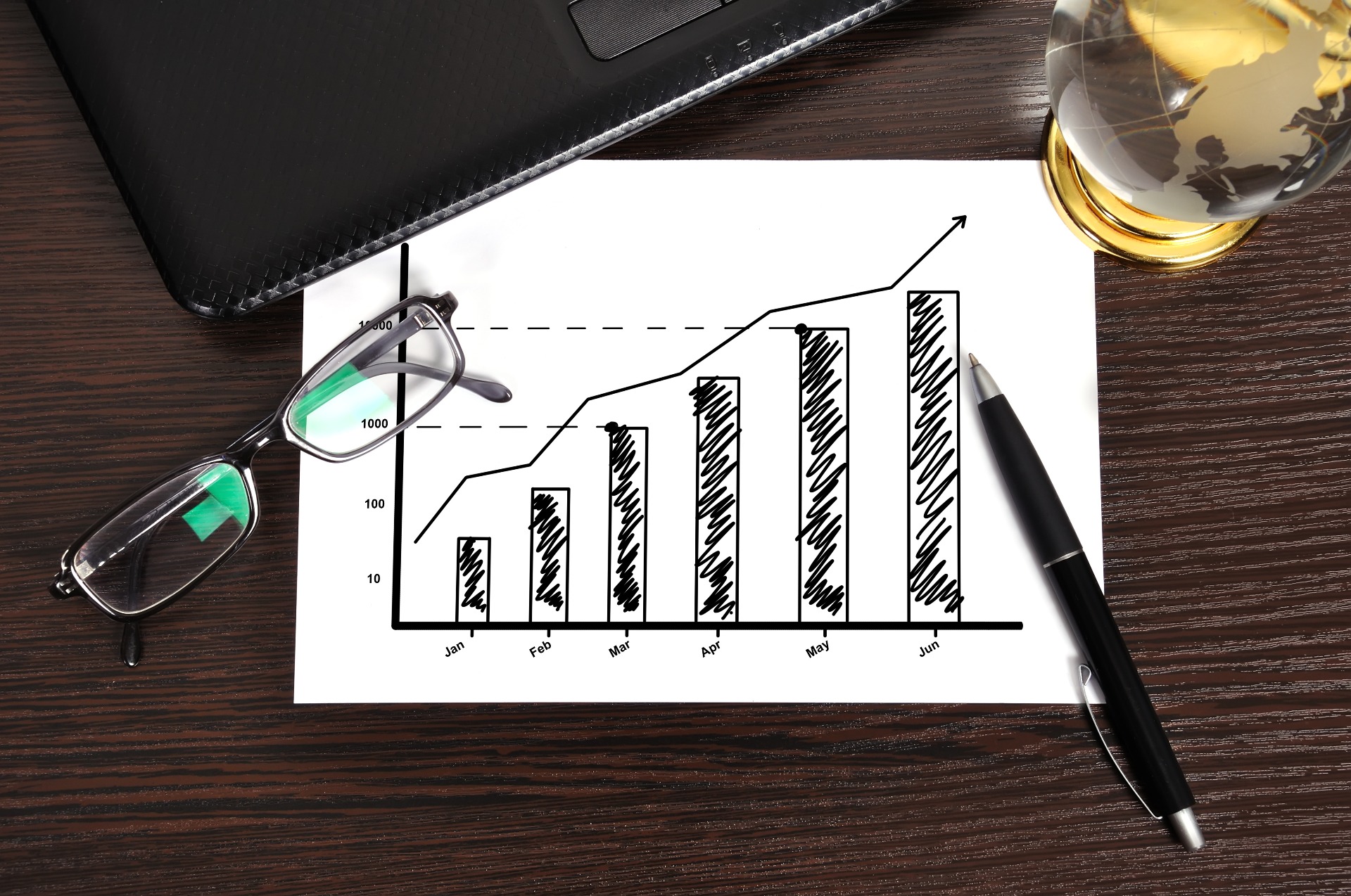
What you'll learn
- Intuition behind probability and statistical analysis
- How to summarize and describe data
- A basic understanding of various methods of evaluating social programs
- How to present results in a compelling and truthful way
- Skills and tools for using R for data analysis
Advanced level
The Challenges of Global Poverty - MITx
What you'll learn
- To identify and analyze some of the root causes of underdevelopment using principles of economics
- To understand the unique constraints and trade-offs the poor face when making decisions
- How to interpret the findings of empirical research that evaluates the effectiveness of anti-poverty strategies, policies, and interventions (including strengths and weaknesses of research)
- A basic understanding of various econometric tools used in development research, which will provide the foundation for participating in more technical courses in development economics
Introductory level
Foundations of Development Policy - MITx
What you'll learn
- Lessons from cutting edge research in development across a range of topics
- How to build and apply economic models relevant to concrete development situations
- How to design and conduct a randomized control trial to learn more about these questions
- Data management and analysis using the software R
Advanced level
Political Economy and Economic Development - MITx
What you'll learn
- Key theories and concepts in political economy and their application to studying economic development.
- Evidence from recent studies on the role of politics and institutions in economic development, and how to interpret their findings.
- Empirical techniques and econometric tools to study political economy and development.
- Data analysis using the software R.
Advanced level
Good Economics for Hard Times - MITx
What you'll learn
- How to use data to tackle some of the toughest problems facing society.
- A more-realistic impression of what current economic research looks like than that typically provided by core introductory microeconomics and macroeconomics courses.
- How modern tools of economists can serve society, what we have already learned and the policy implications, and where important work remains to be done.
Introductory level
Microeconomic Theory and Public Policy - MITx
What you'll learn
- A range of microeconomic theories along with the empirical strategies that have been used to test them
- How to understand and interpret the methodologies and key findings of current microeconomic research
- How to apply microeconomic theory to real-world policy problems facing the US and other advanced economies
Advanced level
Probability - The Science of Uncertainty and Data - MITx
What you'll learn
Skip What you'll learn
- The basic structure and elements of probabilistic models
- Random variables, their distributions, means, and variances
- Probabilistic calculations
- Inference methods
- Laws of large numbers and their applications
- Random processes
Advanced level
Machine Learning with Python: from Linear Models to Deep Learning - MITx
What you'll learn
Skip What you'll learn
- Understand principles behind machine learning problems such as classification, regression, clustering, and reinforcement learning
- Implement and analyze models such as linear models, kernel machines, neural networks, and graphical models
- Choose suitable models for different applications
- Implement and organize machine learning projects, from training, validation, parameter tuning, to feature engineering.
Advanced level
Fundamentals of Statistics - MITx
What you'll learn
- Construct estimators using method of moments and maximum likelihood, and decide how to choose between them
- Quantify uncertainty using confidence intervals and hypothesis testing
- Choose between different models using goodness of fit test
- Make prediction using linear, nonlinear and generalized linear models
- Perform dimension reduction using principal component analysis (PCA)
Advanced level
Data Analysis in Social Science—Assessing Your Knowledge - MITx
What you'll learn
Skip What you'll learn
You will be tested on your knowledge in the following areas:
- Intuition behind probability and statistical analysis
- How to summarize and describe data
- A basic understanding of various methods of evaluating social programs
- How to present results in a compelling and truthful way
- Skills and tools for using R for data analysis
Data Analysis: Statistical Modeling and Computation in Applications - MITx
What you'll learn
Skip What you'll learn
- Model, form hypotheses, perform statistical analysis on real data
- Use dimension reduction techniques such as principal component analysis to visualize high-dimensional data and apply this to genomics data
- Analyze networks (e.g. social networks) and use centrality measures to describe the importance of nodes, and apply this to criminal networks
- Model time series using moving average, autoregressive and other stationary models for forecasting with financial data
- Use Gaussian processes to model environmental data and make predictions
- Communicate analysis results effectively
Advanced level
Statistical Learning with R - Stanford University Online
What you will learn
Skip What you will learn
- Overview of statistical learning
- Linear regression
- Classification
- Resampling methods
- Linear model selection and regularization
- Moving beyond linearity
- Tree-based methods
- Support vector machines
- Deep learning
- Survival modeling
- Unsupervised learning
- Multiple testing
Advanced level
Impact Evaluation Methods with Applications in Low- and Middle-Income Countries - Georgetown University X
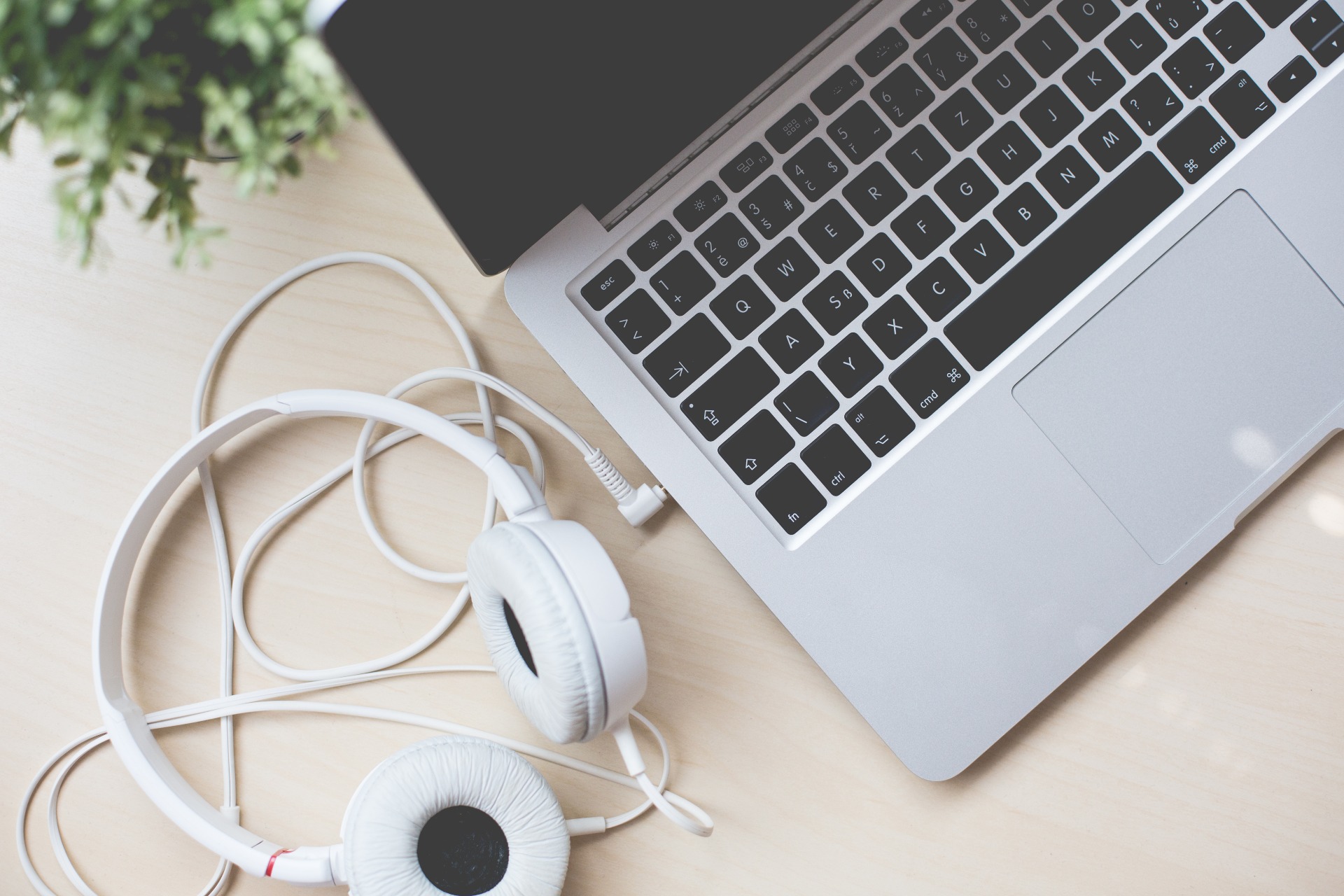
What will you leran?
- Define impact evaluation and recognize its importance.
- Describe the importance of randomization and the problems that can arise in randomized controlled trials (RCTs).
- Identify statistical concepts and tools for program evaluation.
- Interpret the concept of regression and how it informs RCT results.
- Illustrate the balance between sample size and cost of trial.
- Explain the value and application of quasi experimental methods
Introductory level
A Crash Course in Causality: Inferring Causal Effects from Observational Data - University of Pennsylvania
We've all heard the phrase "correlation does not equal causation." So what does equate to causation? This course aims to answer that question and more!
Over the course of 5 weeks, you will learn how causal effects are defined, what assumptions about your data and models are necessary, and how to apply and interpret some popular statistical methods. Students will have the opportunity to apply these methods to example data in R (a free statistical software environment). By the end of the course, students should be able to: 1. Define causal effects using potential outcomes 2. Describe the difference between association and causation 3. Express hypotheses with causal graphs 4. Apply various types of causal inference methods (e.g., matching, instrumental variables, inverse probability of treatment weighting) 5. Identify which causal assumptions are necessary for each type of statistical method So join us... and discover for yourself why modern statistical methods for estimating causal effects are indispensable in so many fields of study!
Introductory level
Causal inference 1 - Columbia University
This course offers a rigorous mathematical study of causal inference at the Master's level. Inferences about causality are of great importance in science, medicine, politics, and business. This course provides an introduction to the statistical literature on causal inference that has emerged over the past 35-40 years and that has revolutionized the way statisticians and applied researchers in many disciplines use data to make inferences about causal relationships.
We will explore methods of data collection to estimate causal relationships. Students will learn to distinguish between relationships that are causal and those that are not; this is not always obvious. Next, we will explore and evaluate the various methods that students can use—such as matching, propensity score subclassification, inverse probability of treatment weighting, and machine learning—to estimate a variety of effects—such as the average treatment effect and the treatment effect on the treated. Finally, we discuss methods for evaluating some of the assumptions we have made, and we offer a preview of extensions that we will address in the sequel to this course.
Intermediate level
Causal inference 2 - Columbia University
This course offers a rigorous mathematical study of advanced topics in causal inference at the master's level. Inferences about causality are of great importance in science, medicine, politics, and business. This course provides an introduction to the statistical literature on causal inference that has emerged over the past 35-40 years and that has revolutionized the way statisticians and applied researchers in many disciplines use data to make inferences about causal relationships.
We will study advanced topics in causal inference, such as mediation, principal stratification, longitudinal causal inference, regression discontinuity, interference, and fixed effects models.
Intermediate model
Econometrics: Methods and applications - Erasmus University Rotterdam
Welcome! Do you want to learn how to analyse and solve business and economic issues with data analysis tools? Then Econometrics from Erasmus University Rotterdam is the right course for you, as you will learn how to translate data into models for forecasting and decision support. * What will I learn? When you know econometrics, you will be able to translate data into models for forecasting and decision support in a wide variety of fields, ranging from macroeconomics to finance and marketing. Our course starts with introductory lectures on simple and multiple regression, followed by topics of special interest covering model specification, endogenous variables, binary choice data and time series data. You will learn these key topics in econometrics by watching the videos with in-video quizzes and by completing training exercises after the video.
* Do I need any prior knowledge? The course is suitable for (advanced) students of economics, finance, business, engineering and data analysis, as well as those working in these fields. The course requires some basic knowledge of matrices, probability and statistics, which are reviewed in the Building Blocks module. If you are looking for a more introductory MOOC on econometrics that requires less prior knowledge of mathematics, you may be interested in the Coursera course "Enjoyable Econometrics", also from Erasmus University Rotterdam * What literature can I consult to support my studies? You can follow the MOOC without studying additional sources. Further reading on the topics covered (including the Building Blocks) can be found in the textbook we have written and on which the MOOC is based: Econometric Methods with Applications in Business and Economics, Oxford University Press. The connection between the modules of the MOOC and the chapters of the book is shown in the Course Guide - More information - How can I continue my studies? * Will there be active teaching assistants to guide me through the course? Staff and PhD students from our Institute of Econometrics will provide guidance in January and February each year. In other periods, we only provide elementary guidance. We always advise you to get in touch with fellow students from this course to discuss topics and exercises. * How will I get a certificate? To obtain the certificate for this course, you are required to complete six test exercises (one per module) and one case project. In addition, you will perform peer review activities on the work of three of your fellow students from this MOOC. You will obtain the certificate if you pass all seven tasks. Have a nice journey through the world of Econometrics! The Econometrics Team
Intermediate level
Probabilistic graphical models 1: Representation - Stanford University
Probabilistic graphical models (PGMs) are a rich framework for encoding probability distributions over complex domains: joint (multivariate) distributions over a large number of interacting random variables. These representations sit at the intersection of statistics and computer science, drawing on concepts from probability theory, graph algorithms, machine learning, and so on. They underlie state-of-the-art methods in a wide variety of applications, such as medical diagnosis, image understanding, speech recognition, natural language processing, and many, many more. They are also a fundamental tool in formulating many machine learning problems.
This course is the first in a sequence of three. It describes the two basic representations of PGMs: Bayesian networks, which are based on a directed graph; and Markov networks, which use an undirected graph. The course discusses both the theoretical properties of these representations and their use in practice. The honors course (highly recommended) contains several practical assignments on how to represent some real-world problems. The course also introduces some important extensions beyond the basic PGM representation, which allow more complex models to be encoded in a compact manner.
Intermediate level
Probabilistic graphical models 2: Inference - Stanford University
Probabilistic graphical models (PGMs) are a rich framework for encoding probability distributions over complex domains: joint (multivariate) distributions over a large number of interacting random variables. These representations sit at the intersection of statistics and computer science, drawing on concepts from probability theory, graph algorithms, machine learning, and others. They underlie state-of-the-art methods in a wide variety of applications, including medical diagnosis, image understanding, speech recognition, natural language processing, and many, many more. They are also a fundamental tool in formulating many machine learning problems.
This course is the second in a sequence of three. Following the first course, which focused on representation, this course addresses the question of probabilistic inference: how a PGM can be used to answer questions. Although a PGM typically describes a very high-dimensional distribution, its structure is designed to allow questions to be answered efficiently. The course presents both exact and approximate algorithms for different types of inference tasks, and discusses where each might best be applied. The honors course (highly recommended) contains two practical programming assignments, in which key routines from the most commonly used exact and approximate algorithms are implemented and applied to a real-world problem.
Intermediate level
Probabilistic graphical models 3: Learning - Stanford University
Probabilistic graphical models (PGMs) are a rich framework for encoding probability distributions over complex domains: joint (multivariate) distributions over a large number of interacting random variables. These representations sit at the intersection of statistics and computer science, drawing on concepts from probability theory, graph algorithms, machine learning, and so on. They underlie state-of-the-art methods in a wide variety of applications, such as medical diagnosis, image understanding, speech recognition, natural language processing, and many, many more. They are also a fundamental tool in formulating many machine learning problems.
This course is the third in a sequence of three. Following the first course, which focused on representation, and the second, which focused on inference, this course addresses the question of learning: how a PGM can be learned from a data set of examples. The course addresses key problems of parameter estimation in both directed and undirected models, as well as the task of learning structures for directed models. The honors course (highly recommended) contains two practical programming assignments, in which key routines from two commonly used learning algorithms are implemented and applied to a real-world problem.
Advanced level
Specialized Program: Methods and Statistics in Social Sciences - University of Amsterdam
This Specialization covers research methods, design, and statistical analysis for social science research questions. In the final Capstone Project, you will apply the skills learned by developing your own research question, collecting data, and analyzing and reporting the results using statistical methods.
Advanced level
Specialized Program: Bayesian Statistics - UC Santa Cruz
This Specialization is aimed at all students who wish to develop their proficiency in statistics, Bayesian statistics, Bayesian inference, R programming, and more. Through four comprehensive courses (From Concept to Data Analysis; Techniques and Models; Mixture Models; Time Series Analysis) and a capstone project, you will cover Bayesian methods—such as conjugate models, MCMC, mixture models, and dynamic linear models—that will provide you with the skills to perform analysis, engage in forecasting, and build statistical models using real-world data.
Applied learning project
This Specializations trains students in the Bayesian approach to statistics, starting with the concept of probability and moving on to more complex concepts such as dynamic linear modeling. They will learn the philosophy of the Bayesian approach, as well as how to apply it to common types of data, and then delve deeper into the analysis of time series data.
Courses in this specialization combine lecture videos, computer-based demonstrations, readings, exercises, and discussion forums to create an active learning experience, while the capstone project is an opportunity for students to demonstrate a broad range of skills and knowledge in Bayesian statistics and apply what they know to real-world data. They will review essential concepts of Bayesian statistics, learn and practice data analysis using R (an open-source and freely available statistical package), perform complex data analysis on a real data set, and write a report on their methods and results.
Advanced level
Narrative economy - Yale University
This course, Narrative Economics, is relatively short and proposes a simple concept: we need to incorporate the contagion of narratives into our economic theory. You can think of narratives as stories that shape the public's beliefs, which in turn influence our decision making. Understanding how people arrived at certain decisions in the past can help our understanding of the economy today and improve our forecasts for the future. Popular thought greatly influences our answers to questions such as how much to invest, how much to spend or save, whether to go to college or take a certain job, and many more. Narrative economics is the study of the viral spread of popular narratives that affect economic behavior. I believe that incorporating these ideas into our research should be done both to improve our ability to anticipate and prepare for economic events and to help us structure economic institutions and policy. Until we better incorporate them into our methods of analysis and forecasting, we will remain blind to a very real, very palpable, and very important mechanism for economic change. Even at the dawn of the Internet and artificial intelligence era, as long as people remain ultimately in control, human narratives will matter. Perhaps they will matter especially as new technology exploits human weaknesses and creates new venues for narrative contagion. Without understanding epidemics of popular narratives, we will not be able to fully understand changes in the economy and economic behavior. The course is divided into 4 modules: Part I introduces the basics and demonstrates how popular stories change over time to affect economic outcomes, including recessions, depressions, and inequality, as well as effective inspiration and growth. These stories can be observed from a variety of sources such as politics, the media, or even popular songs. Part II seeks to answer why some stories go viral while others are quickly forgotten, by more firmly defining our narrative theory. It enumerates and explores a list of seven propositions to help discipline any analysis of economic narratives. Part III examines nine perennial narratives that have demonstrated their ability to influence important economic decisions. These include narratives concerning artificial intelligence, stock market bubbles, and job insecurity. Part IV looks to the future and highlights the opportunities for consilience in the Narrative Economy.We share some thoughts on where narratives are taking us at this point in history and what kind of future research might improve our understanding of them. This course offers only the beginnings of a new idea and some suggestions on how it might be used by economists and financial professionals. The tone is not prescriptive or authoritarian, as my Coursera course, Financial Markets, may be in parts. It represents the beginning of the (epidemic) journey. This course is my way of floating the "germ" of this idea into the broader community of not only practitioners, but anyone interested in finding out how and why things become "important" to us as a society. I hope that some of you will catch this idea, mutate it, spread it, and take it forward. The beginning of the journey is the easy part. The challenge will come in taking these concepts to the next level. We have the tools to incorporate narratives into our research and the moral obligation to act; we just have the work left - Robert J. Shiller
Introductory level